Unlocking Success: The Importance of Image Datasets for Classification
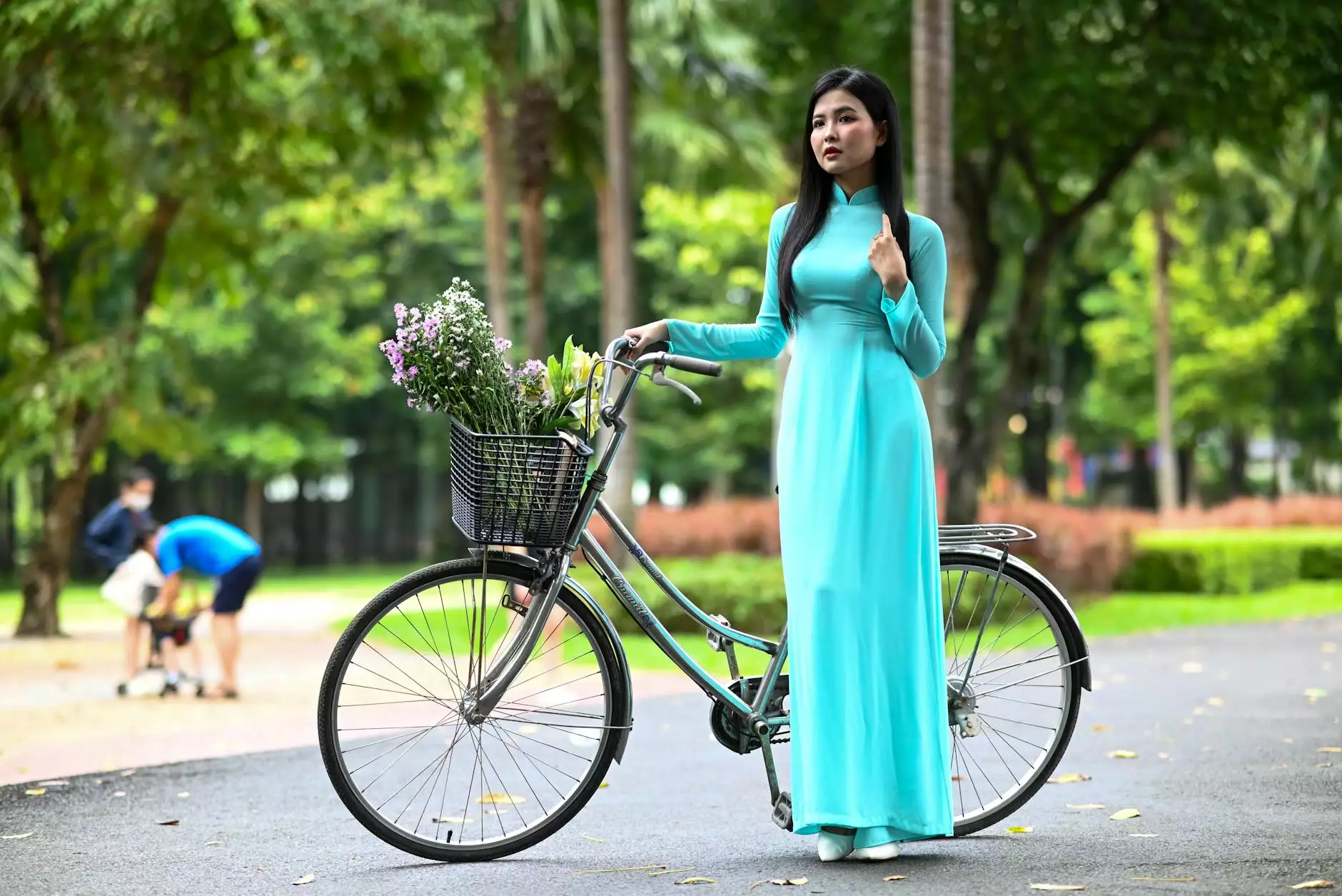
In the current digital landscape, where data drives decisions, the ability to effectively harness and utilize data is paramount. This is particularly true in the realm of image classification, where the quality and accuracy of your datasets can make or break the performance of your machine learning models. In this article, we will explore the significance of image datasets for classification, how they can elevate your business, and how keylabs.ai can provide you with the tools and platforms necessary for success.
The Role of Image Datasets in Machine Learning
Machine learning thrives on data, and the field of computer vision is no exception. Image datasets for classification are collections of images that are annotated and categorized to train and evaluate machine learning models. The primary goal is to teach the models to recognize patterns and make decisions based on visual data.
High-quality datasets are essential for several reasons:
- Improving Accuracy: Well-annotated datasets lead to more accurate predictions.
- Minimizing Bias: Diverse datasets help to reduce bias in model predictions.
- Enhancing Model Performance: Larger and more diverse datasets can significantly enhance the performance of your AI models.
- Facilitating Real-World Applications: Models trained on comprehensive datasets can better generalize to real-world scenarios.
Key Considerations When Choosing Image Datasets for Classification
When selecting or creating your image datasets for classification, it’s crucial to consider various factors to ensure they meet the needs of your project. Here are the main aspects to evaluate:
1. Dataset Quality
The quality of your dataset directly impacts the effectiveness of your machine learning model. Ensure your images are:
- High-resolution: Clear images yield better results.
- Well-annotated: Accurate labels are crucial for supervised learning.
- Diverse: A variety of images ensures robustness in model training.
2. Size and Scale
A larger dataset is generally beneficial, but it should also be relevant. For effective training, aim for:
- Thousands to millions of images: Depending on the complexity of the task.
- Balanced classes: Ensure that each category has enough samples to learn from.
3. Annotation Techniques
There are several methods for annotating images. Choosing the right approach is vital:
- Bounding Boxes: Useful for object detection tasks.
- Segmentation Masks: Essential for pixel-wise classifications.
- Landmarks: Important for tasks involving facial recognition.
Benefits of Utilizing Keylabs.ai for Image Datasets and Data Annotation
As we venture into an era dominated by artificial intelligence, having a reliable partner in data annotation becomes critical. Here’s how keylabs.ai can support your business needs:
Data Annotation Tool
Keylabs.ai offers a state-of-the-art data annotation tool that simplifies the process of creating image datasets for classification. Our platform allows you to efficiently annotate large volumes of images with:
- Easy-to-use Interface: An intuitive design that enhances user experience.
- Collaboration Options: Facilitates teamwork and project management.
- Customizable Annotations: Tailor the annotations to fit your specific needs.
Data Annotation Platform
The keylabs.ai data annotation platform is engineered to support a wide range of machine learning projects. It features:
- Scalability: Easily handle projects of varying sizes.
- Quality Control: Built-in checks ensure high-quality annotations.
- API Integration: Seamlessly integrate with your existing workflows.
Best Practices for Working with Image Datasets for Classification
When it comes to utilizing image datasets for classification, adherence to best practices can make a substantial difference:
1. Preprocessing Your Images
Before training your model, proper preprocessing is critical. Consider:
- Normalizing: Ensuring consistency in image data.
- Augmenting: Using techniques like rotation, scaling, and flipping to expand your dataset.
2. Splitting Your Dataset
To measure the effectiveness of your model, divide your dataset into:
- Training Set: The primary set used to train your model.
- Validation Set: For tuning model parameters.
- Test Set: To evaluate the model’s performance on unseen data.
3. Continuous Learning and Feedback
Machine learning is an iterative process. Collect feedback based on model performance, and refine your datasets and annotations accordingly.
Conclusion: Empowering Your Business with Quality Data
In the fast-evolving world of technology, leveraging image datasets for classification is not just an option; it’s a necessity for businesses aiming to thrive. With platforms like keylabs.ai, companies can access superior data annotation tools that enhance data quality and drive model efficacy.
By prioritizing high-quality datasets, understanding the nuances of annotation techniques, and adhering to industry best practices, your organization can position itself at the forefront of innovation. At keylabs.ai, we are committed to providing you with the tools and support necessary to unlock the full potential of your image classification projects.
Explore our offerings today, and take the first step toward transforming your business with robust image classification capabilities. Choose quality, choose keylabs.ai.