Mastering Image Datasets for Object Detection
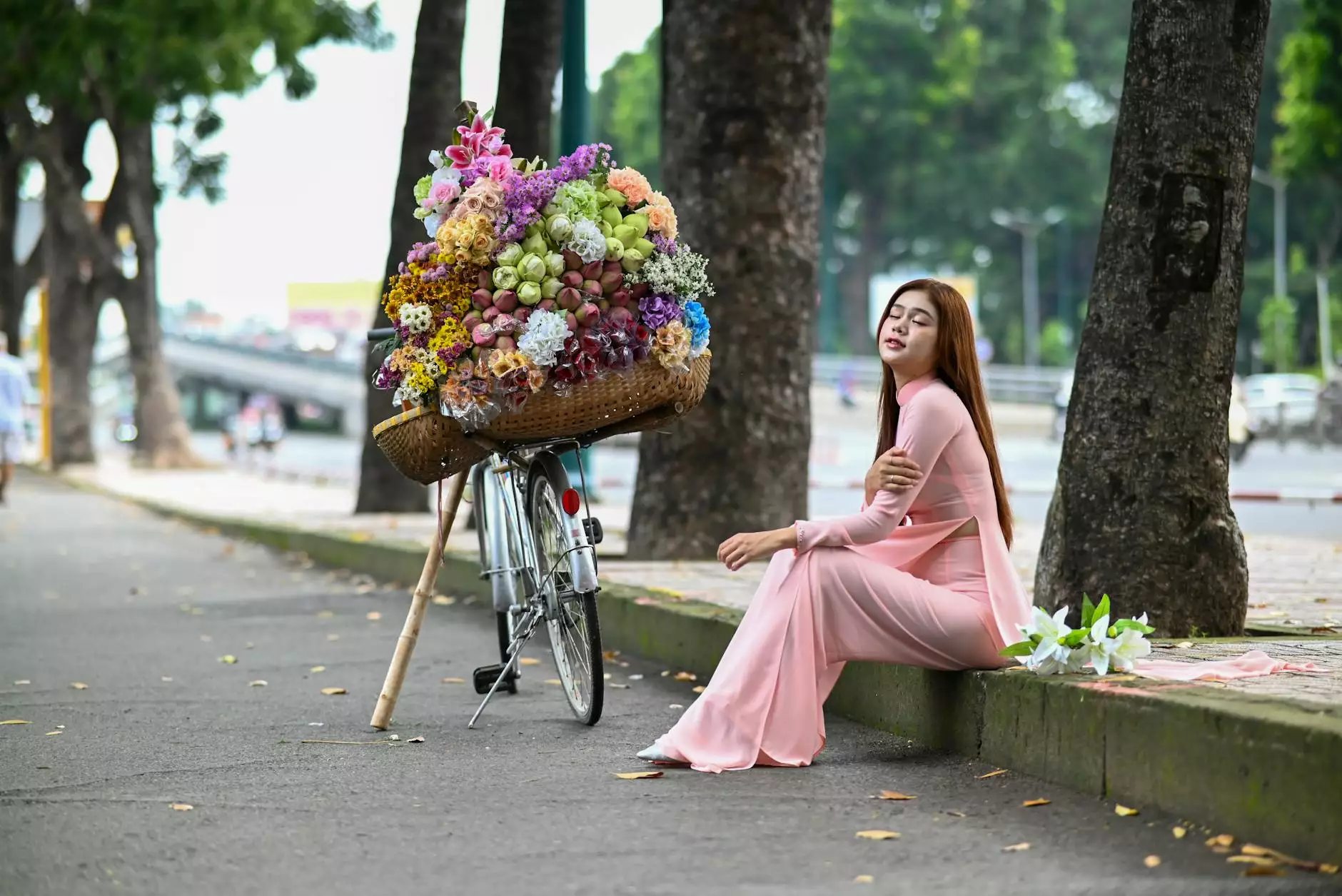
In today’s fast-paced technological world, the demand for advanced image recognition and object detection has surged. As businesses move towards leveraging AI-driven solutions, understanding the importance of an image dataset for object detection cannot be overstated. This article will delve into the intricacies of creating and utilizing image datasets, the significance of data annotation tools and platforms, and how organizations like Keylabs.ai are leading the charge in this transformative space.
1. The Significance of Image Datasets in Object Detection
Object detection is a crucial aspect of computer vision that involves identifying and localizing objects within an image. To build a robust object detection model, extensive and high-quality image datasets are essential. These datasets act as the backbone of machine learning algorithms, providing the necessary data for training, validating, and testing models.
1.1 Components of Image Datasets
An effective image dataset for object detection consists of:
- Diverse Image Selection: A wide variety of images representing real-life conditions helps models generalize better.
- Annotation Quality: Each image must be accurately annotated, indicating the presence and location of objects.
- Balanced Class Distribution: Ensuring that all classes of objects are evenly represented prevents bias in the model.
2. Data Annotation: The Heart of Object Detection
Data annotation is the process of labeling images to provide essential information for training machine learning models. It is a meticulous task that requires attention to detail. The quality of annotations directly influences the performance of object detection systems.
2.1 Types of Annotations
The following types of annotations are commonly used in object detection:
- Bounding Boxes: These are rectangular boxes drawn around objects to indicate their position.
- Segmentation Masks: These provide pixel-level annotations for more precise identification of object boundaries.
- Keypoint Annotations: Used mainly for human poses, these indicate specific points of interest on an object.
3. Choosing the Right Data Annotation Tool
Businesses looking to create effective image datasets need reliable data annotation tools. The right tool can significantly enhance productivity and accuracy in the annotation process.
3.1 Features of Effective Data Annotation Tools
When selecting a data annotation tool, consider the following features:
- User-Friendly Interface: A tool that is easy to navigate allows for quicker and more efficient annotation.
- Collaboration Capabilities: Enables teams to work together seamlessly, ensuring consistent annotation standards.
- Integration with Machine Learning Frameworks: Facilitates a smoother transition from data preparation to model training.
- Support for Various Annotation Types: Having flexibility in types of annotations makes the tool versatile for different projects.
4. Building a Comprehensive Image Dataset
Creating an image dataset for object detection involves several critical steps:
4.1 Step 1: Collecting Images
The first step is to gather a comprehensive collection of images. Sources can include:
- Company owned resources
- Open-source image repositories
- Crowdsourcing platforms
It's crucial to ensure a diverse range of images to cover different scenarios and variations of objects.
4.2 Step 2: Annotating the Images
Utilize a reliable data annotation tool toannotate the images, ensuring that every object of interest is accurately labeled. Quality control during this stage is vital; review annotations for accuracy and consistency.
4.3 Step 3: Validating the Dataset
Validation ensures the dataset meets the required standards. This may involve random sampling of images for manual review or utilizing automated checks within the annotation tool.
4.4 Step 4: Organizing the Data
Organize the dataset into folders by categories or classes, making it easier for machine learning models to access and process the data efficiently.
5. The Role of Keylabs.ai in Data Annotation
Keylabs.ai is at the forefront of providing powerful data annotation platforms that empower businesses to create high-quality image datasets for object detection.
5.1 Innovative Solutions Tailored for Businesses
With a cutting-edge data annotation tool, Keylabs.ai offers:
- Scalability: Easily scale your annotation projects as your needs grow.
- Expert Annotators: Access to a team of skilled annotators who ensure high accuracy in labeling.
- Customizable Workflows: Adapt the annotation process to fit specific project requirements.
6. Leveraging Data Annotation Platforms for Better Outcomes
By utilizing data annotation platforms like Keylabs.ai, businesses can enhance their operational efficiency and drive better outcomes:
6.1 Accelerated Project Timelines
With efficient annotation processes and a dedicated team, projects can be completed faster, allowing companies to bring their object detection solutions to market quickly.
6.2 Improved Model Accuracy
High-quality annotated data leads to better-trained models, which directly impacts the accuracy and reliability of object detection systems.
6.3 Cost-Effectiveness
Outsourcing data annotation reduces overhead costs associated with in-house annotation teams while promoting access to specialized skills.
7. Best Practices for Working with Image Datasets
To maximize the effectiveness of image datasets for object detection, consider the following best practices:
7.1 Regularly Update Your Dataset
Image datasets should be dynamic. Regular updates help in accommodating new objects and scenarios, enhancing the robustness of detection models.
7.2 Ensure Ethical Data Usage
Be mindful of copyright and privacy issues associated with images. Use publicly available datasets or obtain permission when necessary.
7.3 Leverage Community Resources
Join forums and communities focused on computer vision and object detection to share insights, datasets, and tools. Collaboration often leads to richer datasets and better results.
8. The Future of Object Detection and Image Datasets
As AI and machine learning technologies evolve, so too will the methods of collecting and annotating image datasets. Emerging techniques such as automated annotation using AI and synthetic data generation are set to revolutionize how datasets are built.
8.1 Automated Annotation Tools
Leveraging AI to automate parts of the annotation process can significantly reduce time and resources required for data preparation.
8.2 Synthetic Datasets
The use of synthetic data generated from simulations is becoming increasingly popular. This approach allows the creation of diverse datasets that may be difficult to capture in the real world.
Conclusion
In summary, obtaining and managing a high-quality image dataset for object detection is an essential element for any business looking to implement effective AI-driven solutions. By employing reliable data annotation tools and adhering to best practices, organizations can significantly enhance their object detection capabilities. Companies like Keylabs.ai provide invaluable resources and expertise to make this process smoother and more efficient. As the landscape of object detection evolves, staying informed and adaptable will be vital for businesses aiming to leverage these technologies to their fullest potential.